|

|

|

|

|
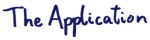
|
Supply chain planning for a manufacturer of polyester yarn. Molten polyester (PET) is extruded
through a "spinneret" (something like a shower head) to produce multiple strands – up to
300. The strands from one spinneret are cooled, oiled, rolled, stretched and twisted together to
form a single filament, which is wound onto a reel. The product grade is determined by the choice
of spinneret (number of holes and diameter), the temperature and flow rate, and additives in the PET.
|
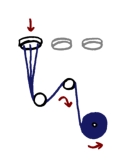
|
Spinnerets are normally grouped into 6, 8 or 12 in one "position" and all the spinnerets
in one position make the same product. Positions are grouped into "housings" and housings
into "groups", and finally a production line is normally made up of two groups. In
total, there are normally 36 positions in a production line. In this application, there are over
30 spinning lines.
Each line has a subset of products that it is able to make, and preferences based on quality within
that subset. Changeovers involving a change of spinneret are expensive, because of the time taken
to refit, and because the spinnerets cannot be reused once removed. (The metal is recycled.)
|
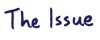
Most of the lines are capable of making multiple products at one time, though there is a practical
limit of three products at once. There are complex restrictions on which products can run together,
arising from feasible temperature and flow rate bands, which are controlled at different levels in the
equipment hierarchy.
|
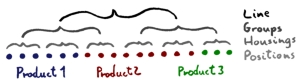
|
Modelling these restrictions is too complex to discuss here. A more fundamental question is
how to model spinneret changeovers without having to model individual positions.
|
If we do not model the changeovers, the cost cannot be recognised, and the optimal solution may contain
an unacceptable variability of products on some lines. If we model individual positions, we get a huge
number of zero-one variables representing the decision to make each product at each position in each time
period (weeks and months), and the model becomes impossible to solve.
That is not the end of the problems – standard changeover modelling simply counts the number of
products in each period and ignores the benefit of continuing to make the same product over the time period
end. (This is because you then need to be concerned about which product is first and last in each
period, which adds a level of complexity.)
|

We use an extra piece of information. Changeovers are such a large part of the cost that most of
the time a position will run the same product for at least a time period and often more than one. This
makes it (just about) reasonable to assume that changeovers will only happen at period ends. Instead
of assigning products to individual positions, we can just calculate the number of positions needed to make
the planned quantity over the time available.

Production rate and period length are constants, so this is a linear relationship between quantity of
product and number of positions. Number of positions is defined as a continuous variable, so two
and a half positions is possible. This is nonsensical, but forcing the variable to take integer
values would over-restrict the possible production levels.
|
We need to derive the number of changeovers. Suppose 6 positions make product 1 in the first
period, and 8 positions make product 1 in the next. Then at the very least, two positions must
change over from whatever they were making in the first period at the start of the next. This is
just a linear constraint:
|
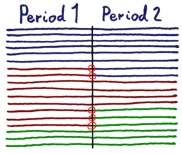
|
We apply the changeover costs to this minimum value and accumulate over all products. The net
result is that we have a fairly inaccurate but very computationally cheap measure of the total cost of
changeovers. We should not expect to be more accurate in a model with long time periods. More
important than accuracy, we can now penalise changes in product mix on each line over time, so steady
production is encouraged. The optimal solution provides a good starting point for detailed daily
scheduling.
|
|